Shape Skeleton Transformation Tools
A dataset for evaluating one-shot categorization of novel object classes
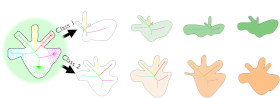
The following code shows how to create shape variants by manipulating a shape's skeletal representation. The skeletal representation decomposes the shape into parts. Here we vary the relationship of the lengths, orientations, widths, and positions of all these parts to create new shape variants.
Such new variants may be useful in producing stimuli for a variety of psychophysical tasks. As an example, the code can be used to create classes of objects with similar/dissimilar statistics (e.g., by choosing the distribution of parameters of how these parts are sampled).
Related Papers
- Morgenstern, Y., Hartmann, F., Schmidt, F., Tiedemann, H., Prokott, E., Maiello, G., & Fleming, R. W. (2021). An image-computable model of human visual shape similarity. PLOS Computational Biology, 17(6), e1008981. https://doi.org/10.1371/journal.pcbi.1008981
- Morgenstern, Y., Schmidt, F., & Fleming, R. W. (2020). A dataset for evaluating one-shot categorization of novel object classes. Data in Brief, 105302. https://doi.org/10.1016/j.dib.2020.105302
- Morgenstern, Y., Schmidt, F., & Fleming, R. W. (2019). One-shot categorization of novel object classes in humans. Vision Research, 165, 98-108.
Liquids
Sets of high-quality liquid animations and machine learning sets.
Observers are remarkably good at visually inferring the viscosity of flowing fluids. They use multiple midlevel shape and motion features to do so. How they choose, identify, compute and combine these features is an important question.
Here, we provide two smaller sets of high-quality renderings of 10s liquid simulations (and corresponding perceptual data) together with another massive set of 20.000 lower-quality renderings of 0.67s liquid simulations (and corresponding trained deep neural networks and perceptual data), optimized for machine learning purposes. The data can be used to investigate visual perception of liquids as well as (machine learning) models of liquid viscosity from dynamic animations and static images.
Related Papers
- van Assen, J. J. R., Nishida, S. Y., & Fleming, R. W. (2020). Visual perception of liquids: Insights from deep neural networks. PLOS Computational Biology, 16(8), e1008018.
- Van Assen, J. J. R., Barla, P., & Fleming, R. W. (2018). Visual features in the perception of liquids. Current biology, 28(3), 452-458.
- van Assen, J. J. R., & Fleming, R. W. (2016). Influence of optical material properties on the perception of liquids. Journal of vision, 16(15), 12-12.